Three frameworks for AI model safety designations
Could AI safety evals learn from FDA approvals, NYC restaurant inspection grades, or Energy Star-designated appliances?
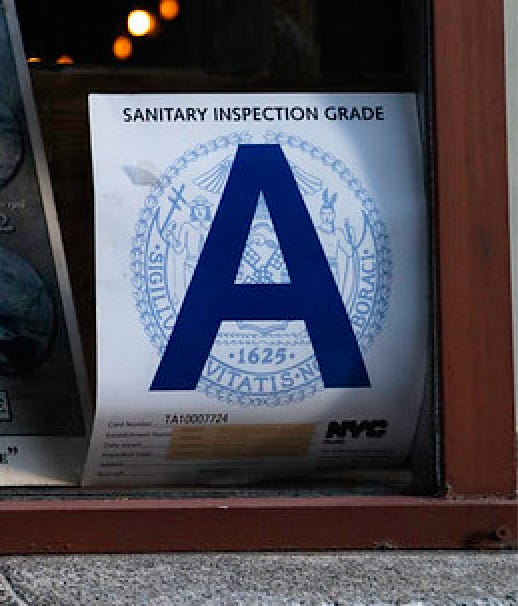
One major challenge in AI governance is the lack of audit and safety standards—particularly by a trustworthy third-party, e.g. not from a foundation model developer or investor.
It’s been on the AI policy agenda for several years: In 2022, audit design was the subject of a $71k competition at Stanford Human-Centered Artificial Intelligence (HAI), which challenged participants to build tools to assess AI systems for bias or discrimination potential. And in April 2024, researchers at Technion and the Transformative Futures Institute argued for the establishment of an AI Audit Standards Board—a technically-savvy body that would keep AI evaluation processes relevant and responsive to changes in the industry.
As the pace of AI research accelerates faster than policymakers can keep up with, it’s important that nonprofit and government agencies figure out a way to test generative models for safe use. Just as important is effective, resonant messaging, given that public perceptions of trustworthiness and competence rank lower for government and media than for private sector companies and leaders, according to the 2024 Edelman Trust Barometer.
So, what could it look like for a third-party evaluator to establish meaningful criteria for AI model safety — and issue a stamp of approval for broad use of foundation models? And what would it take to get the general public to trust and rely on such a framework?
In this post, I draw inspiration from three familiar safety and regulatory compliance programs:
FDA approvals
NYC restaurant inspection grades
Energy Star-designated appliances
FDA approvals
The U.S. Food and Drug Administration (FDA) stands out as an example of a regulatory body that generally garners trust and respect — it’s a green or red light for what’s safe for human consumption. In the case of drug approval, FDA’s process entails substantial analysis of risks and benefits, clinical trial data, and existing treatments. In unusually high-priority cases, FDA has three special designations for drug development and review—Fast Track, Breakthrough Therapy, and Priority Review—in addition to an Accelerated Approval procedure to advance treatments for life-threatening conditions.
A standardized yet adaptable AI audit process could draw inspiration from FDA drug approvals, provided a U.S. government body could determine and make clear its position on AI safety. In other words, FDA approvals help us decide what drugs and supplements to take or avoid; they also inform product commercialization, since drugs have to be FDA-approved in order to be marketed in the U.S. But it’s easier to point to side effects and death risks in medications than it is in AI models.
It also doesn’t have to be as simple as using the FDA as overarching inspiration for AI policy. This January, the AI Now Institute facilitated a conversation about an ‘FDA for AI’ with former government officials, academics, doctors, lawyers, computer scientists, and journalists—one key takeaway: policymakers might take inspiration from FDA-style interventions across the AI supply chain, not necessarily the entire agency structure itself.
Another specific takeaway from the FDA/AI conversation: consider regular, externally monitored audits with publicly available results for AI models, just like the FDA does to ensure continuous compliance in the pharmaceuticals industry (Manheim et al. 2024, 1.2).
NYC restaurant inspection grades
Letter grades hit close to home for myriad alumni of the A-F grading scale. That’s why the New York City Department of Health’s restaurant inspection program has sent such a clear signal with its Scarlet Letter-like sanitation grade. The main idea: NYC DOH inspectors routinely evaluate the city’s ~27K restaurants for compliance with food safety regulations, tally violations into a total score, and assign a standardized, corresponding letter grade: “A” for 0-13 points, “B” for 14-27 points, and “C” for 28+ sanitary violation points. Fewer points means a higher letter grade — and restaurants must post them for patrons to see.
Rather than a blanket stamp of approval, an understandable and explainable score range or grading framework balances transparency with consumer choice: enter at your own risk. Perhaps there’s room for even more nuance than an overall letter grade, too. Take the case of the MLCommons AI Safety benchmark proof-of-concept—announced this April, the global working group’s community-developed scoring mechanism assigns a rating for each of several major hazards, including hate, violent crimes, weapons of mass destruction, self-harm, and more. Instead of an A-F (or for NYC restaurants, A-C) scale, MLCommons ranges from high risk (H) to moderate-high risk (M-H), moderate risk (M), moderate-low risk (M-L), and low risk (L). Importantly, AI-based safety risks differ in severity and urgency level enough that it’s smart to evaluate and score by individual risk rather than provide an overall score.
Energy Star-designated appliances
Over 30 years old, the U.S. Department of Energy (DOE) and Environmental Protection Agency (EPA)’s Energy Star program sets an energy efficiency standard for household appliances—originally intended for computer monitors, Energy Star today covers everything from air conditioners and dishwashers to washers and dryers. Products that meet the federal government’s energy efficiency criteria and pass a third-party inspection can go to market with a recognizable blue sticker, indicating approval from the Energy Star program.
Since 1992, Energy Star has prevented 4 billion metric tons of greenhouse gas emissions from entering the atmosphere, and has saved U.S. households and businesses more than $500 billion in energy costs, the EPA says. It’s well-recognized, too: ~90% of American households are familiar with Energy Star. But it’s not without its critics: a 2010 Government Accountability Office (GAO) report found that the Energy Star certification was vulnerable to fraud and abuse—it was easy for bogus products to get certified. Today, a third-party evaluation process aims to address that.
Imagine if AI models met government-aligned standards, passed a third-party evaluation process, and could go to market with a special certification and insignia. Instead of a letter grade or a more rigid approval to market, a safety certification process could provide extra assurances in the market without getting too wonky at face value — though AI is a market where there’s surely appetite for transparent, detailed evaluations.
Toward a future with AI safety standards
FDA drug approvals, NYC restaurant inspection grades, and the blue Energy Star sticker illustrate three trustworthy frameworks for evaluation and approval in regulated industries. But that’s the kicker — regulation in AI is nascent and minimal, due in large part to a gap in technical prowess and pace between developers and policymakers.
While these frameworks offer inspiration for broadly recognizable and trustworthy AI safety standards, it’ll be important to implement them in such a way that balances the proprietary vs. open-source debate. Too much influence from a small handful of organizations might mean that models from the biggest tech companies more quickly get a stamp of approval, making it harder to meaningfully evaluate small and up-and-coming generative models. At the same rate, larger model providers have much to explain on data privacy, let alone other societal concerns like bias. And developers of powerful open-source models have their work cut out for them: explaining why policymakers needn’t be concerned about technology that is both capable and modifiable.